To honor the life of Daniel Kahneman, this week’s post is on his final book, Noise.
Noise unpacks the variability in judgements leftover once bias is removed. Judgements take many forms, such as which projects to pursue or whom to hire. While there is a litany of research and practical techniques to reduce bias in society, surprisingly little attention is paid to noise. A crucial problem of noise is that, contrary to intuition, it’s additive rather than offsetting. For example, if you overprice one business deal and underprice another, it’s useless to say that you got it right on average. The former will turn out to be a poor investment, and there’s a good chance you’ll miss out on the latter.
To reduce noise, Daniel, Olivier and Cass recommend “decision hygiene” techniques. These include carefully selecting a well-qualified and diverse set of experts to opine, ensuring that their positions are made independently, and using simple algorithms to aggregate viewpoints. The key is to focus on improving the decision making process rather the outcome of the decision itself. The most complicated judgements are handled effectively when decision makers remain open to new information and actively seek contradictory views to evolve their hypothesis.
This book has given me a set of tools and idea to improve my broader team’s and my own judgements when making challenging subjective decisions.
You should read this book if you…
- want to improvement you and/or your teams’ judgements
- seek to understand the various sources of noise and how to reduce it
- want to know when it’s okay to accept variability in judgements
Additional Information
Year Published: 2021
Book Ranking (from 1-10): 8 – Very Good – In depth insights on a specific topic
Ease of Read (from 1-5): 4 – Moderately challenging
Key Highlights
- To understand error in judgment, we must understand both bias and noise. Sometimes, as we will see, noise is the more important problem. But in public conversations about human error and in organizations all over the world, noise is rarely recognized. Bias is the star of the show. Noise is a bit player, usually offstage. The topic of bias has been discussed in thousands of scientific articles and dozens of popular books, few of which even mention the issue of noise. This book is our attempt to redress the balance
- Matters of taste and competitive settings all pose interesting problems of judgment. But our focus is on judgments in which variability is undesirable. System noise is a problem of systems, which are organizations, not markets. When traders make different assessments of the value of a stock, some of them will make money, and others will not. Disagreements make markets. But if one of those traders is randomly chosen to make that assessment on behalf of her firm, and if we find out that her colleagues in the same firm would produce very different assessments, then the firm faces system noise, and that is a problem
- A frequent misconception about unwanted variability in judgments is that it doesn’t matter, because random errors supposedly cancel one another out. Certainly, positive and negative errors in a judgment about the same case will tend to cancel one another out, and we will discuss in detail how this property can be used to reduce noise. But noisy systems do not make multiple judgments of the same case. They make noisy judgments of different cases. If one insurance policy is overpriced and another is underpriced, pricing may on average look right, but the insurance company has made two costly errors. If two felons who both should be sentenced to five years in prison receive sentences of three years and seven years, justice has not, on average, been done. In noisy systems, errors do not cancel out. They add up
- Judgment can therefore be described as measurement in which the instrument is a human mind. Implicit in the notion of measurement is the goal of accuracy—to approach truth and minimize error. The goal of judgment is not to impress, not to take a stand, not to persuade
- Scholars of decision-making offer clear advice to resolve this tension: focus on the process, not on the outcome of a single case. We recognize, however, that this is not standard practice in real life. Professionals are usually evaluated on how closely their judgments match verifiable outcomes, and if you ask them what they aim for in their judgments, a close match is what they will answer
- To summarize, we discussed several types of noise. System noise is undesirable variability in the judgments of the same case by multiple individuals. We have identified its two major components, which can be separated when the same individuals evaluate multiple cases: Level noise is variability in the average level of judgments by different judges. Pattern noise is variability in judges’ responses to particular cases
- A simple choice between procedures: if you can get independent opinions from others, do it—this real wisdom of crowds is highly likely to improve your judgment. If you cannot, make the same judgment yourself a second time to create an “inner crowd.” You can do this either after some time has passed—giving yourself distance from your first opinion—or by actively trying to argue against yourself to find another perspective on the problem. Finally, regardless of the type of crowd, unless you have very strong reasons to put more weight on one of the estimates, your best bet is to average them
- Recall the basic finding of group polarization: after people talk with one another, they typically end up at a more extreme point in line with their original inclinations
- Equal-weight models do well because they are not susceptible to accidents of sampling
- Because of confirmation bias and desirability bias, we will tend to collect and interpret evidence selectively to favor a judgment that, respectively, we already believe or wish to be true
- The only cognitive style that predicts forecasting ability was ‘actively open-minded thinking’, which means to actively search for information that contradicts your preexisting hypotheses. Such information includes the dissenting opinions of others and the careful weighing of new evidence against old beliefs. This, however, goes beyond slow and careful thinking. It is the humility of being constantly aware that your judgment is a work in progress and a yearning to be corrected
- Apart from general intelligence, we could reasonably expect that superforecasters are unusually good with numbers. And they are. But their real advantage is not their talent at math; it is their ease in thinking analytically and probabilistically
- To characterize the thinking style of superforecasters, Tetlock uses the phrase “perpetual beta,” a term used by computer programmers for a program that is not meant to be released in a final version but that is endlessly used, analyzed, and improved
- The upshot is that a system that depends on relative evaluations is appropriate only if an organization cares about relative performance
- We have defined noise as unwanted variability, and if something is unwanted, it should probably be eliminated. But the analysis is more complicated and more interesting than that. Noise may be unwanted, other things being equal. But other things might not be equal, and the costs of eliminating noise might exceed the benefits. And even when an analysis of costs and benefits suggests that noise is costly, eliminating it might produce a range of awful or even unacceptable consequences for both public and private institutions
- Because rules have clear edges, people can evade them by engaging in conduct that is technically exempted but that creates the same or analogous harms. (Every parent of a teenager knows this!) When we cannot easily design rules that ban all conduct that ought to be prohibited, we have a distinctive reason to tolerate noise, or so the objection goes
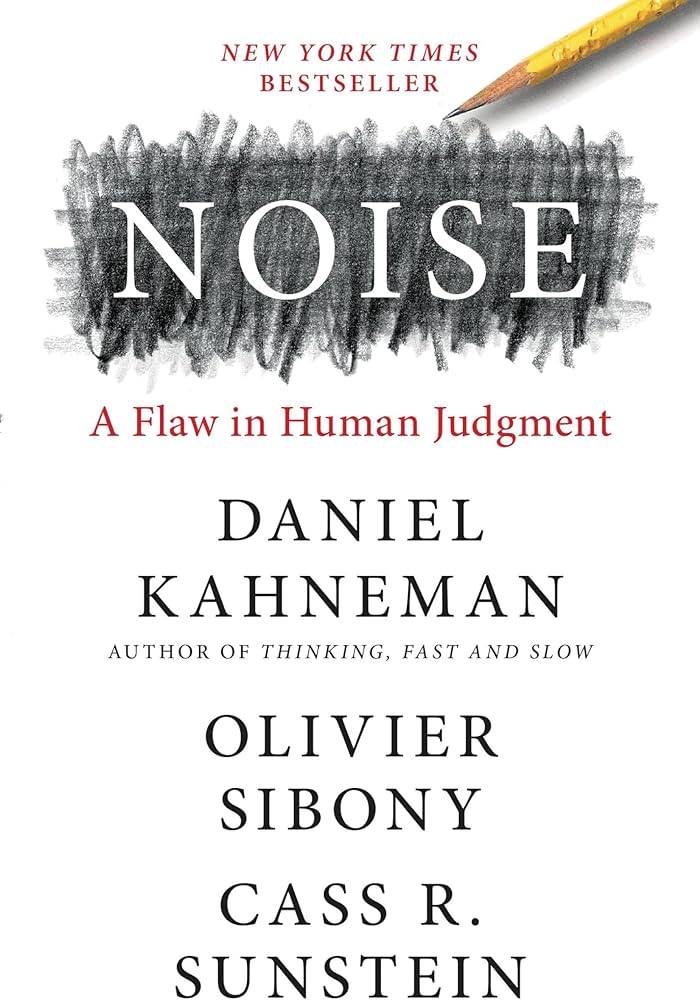
Discover more from The Broader Application
Subscribe to get the latest posts sent to your email.